How to setup a customer churn prediction use case
If you’re working in sales or marketing and are interested in integrating an AI churn model into your processes, we have a comprehensive step-by-step guide to help you do so successfully.
By implementing a churn model you can improve customer lifetime value with 30%
Integrating processes between business and IT is key for success and value realization. And don't forget to celebrate with cake!
The Churn Use Case
Hello Future: Implementing a customer churn prediction model and making your customers stay!
In the world of sales and marketing, finding new ways to keep customers is very important. As we have heard, it can be anywhere from 5-25 times as expensive to gain a new customer versus taking care of your existing ones. Leveraging data and using Artificial Intelligence (AI) as part of your customer journeys to predict customer churn and prevent your customers from leaving is a great way to do this, especially if you are in a subscription-based business. In this article, we dig into the concept of customer churn prediction – understanding why customers leave – and look at what it takes to make better decisions by implementing a churn model. This article is part of an educational concept that we call “Hello Future”, where we at the consulting company Deerdata aim to address AI and automation from a practical business perspective. This article will help you understand how to use AI to keep your customers and get started with customer churn prediction.
Our Hello Future use case courses and articles go through the following steps.
- Value and how to measure impact
- Steps in the process
- Roles and skills
- Data
- System(s)
And no use case is complete without some deer advice from the DeerData team. So, let’s dig into customer churn prediction!

Picture 1: The Deerdata training framework
1. The Value of a Churn Model: A Game-Changer for Your Business
Imagine a world where your customers rarely leave, and if they show signs of departure, you’re already a step ahead, ready to re-engage. That’s the power of customer churn prediction. Traditionally, businesses operate on rule-based engagement – welcome messages, content updates, and goodbye notes. In this article, we will use a fictive company called Deerdata TV – a subscription-based streaming service as an example company to start exemplifying. Below you see an example of Deerdata TV’s rule-based customer journeys.
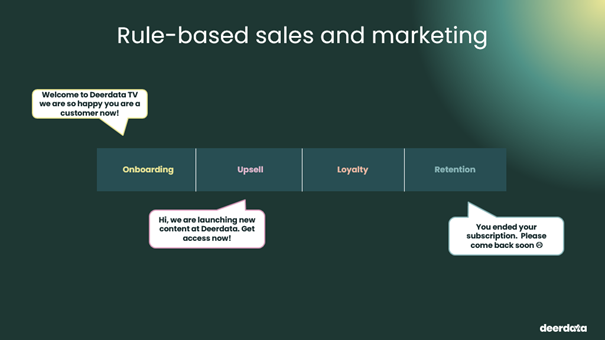
Picture 2: Rule based marketing
However, integrating a churn model elevates this approach, allowing for proactive engagement with at-risk customers through targeted incentives like a free subscription month or exclusive content previews. See the examples below in picture 3. Sounds like a big step? Don’t worry, it is not about starting from scratch but enhancing existing marketing and sales journeys with sharper, data-driven intelligence so you can address your customer in an even more timely manner. Like this, you can take iterative steps and evolve your process gradually to an ecosystem that is combining rules and more advanced analytics. And remember, automation is key. In order to scale, you have to reduce the manual work and automate where you can.
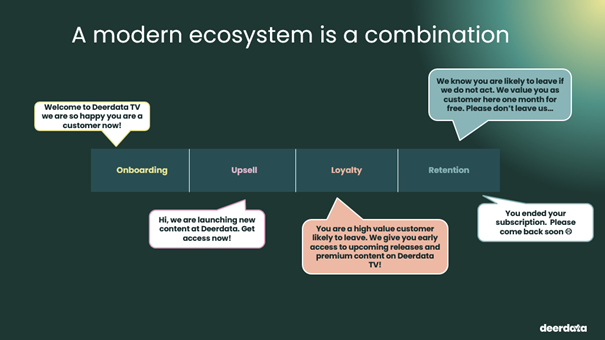
Picture 3: A combination of rules and AI in your eco-systems
When deciding to embark on a journey around implementing AI in your processes, value is key. In the above case, how would you know it is adding value to your ecosystem? Well, you do not. You need to measure it. And to measure, you need a baseline through well-defined KPIs, such as the churn rate, customer lifetime value, and customer satisfaction. Just measuring churn will not be enough as you have to balance how much to give up to retain customers; complementing this with customer lifetime value is a good way to balance between volume and value. There are many other KPIs you can use but independent of what you choose, remember that being able to show and prove value continuously when implementing AI is key in driving change and getting key stakeholders on board.
2. Steps in the process: From Concept to Value Realization
So where to start and what are the key steps to not just build the model but deliver value to the business? Remember this is key. Just building an AI model that is not well aligned with business needs and that does not integrate well into existing processes is not going to add any value and is likely to end up in the proof-of-concept graveyard (trust me, I have been there….)
A quick overview of the key steps:
- Aligning Business Requirements and Stakeholders: It all starts with clarity on your goals and getting everyone on board.
- Data Discovery: The quality and accessibility of your data are foundational to moving forward.
- Model Development: AI is not magic but mathematics. Choosing the right algorithm to predict churn is vital.
- Deployment and Integration: Once the model is ready, it’s about weaving it into your existing system landscape.
- Continuous Evaluation: The work doesn’t stop at deployment. Continuously tracking performance and value realization is key.
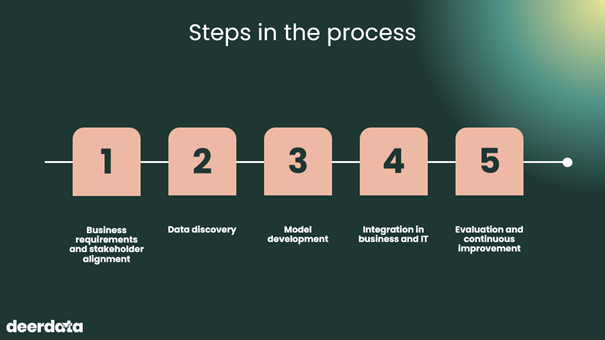
Picture 4: Steps in the process – from concept to value realization
3. Roles and Skills: The Team Behind the Magic
Do the above steps feel like an impossibility? Don’t worry no one has all these steps figured out. Implementing a churn model is a team sport, requiring a mix of business acumen and technical expertise. The dream team includes the following roles:
- Data Engineers: The architects of your data infrastructure.
- Data Scientists: The brains behind the model, constantly refining and improving its accuracy.
- Business Analysts: The bridge between business needs and technical solutions.
- Business Owners: The visionaries who see the model’s potential to transform customer retention.
- End Users: The frontline warriors, leveraging the model to make data-driven decisions.
These roles all work together and if you have not already check out the concept of cross functional teams, I highly recommend it.
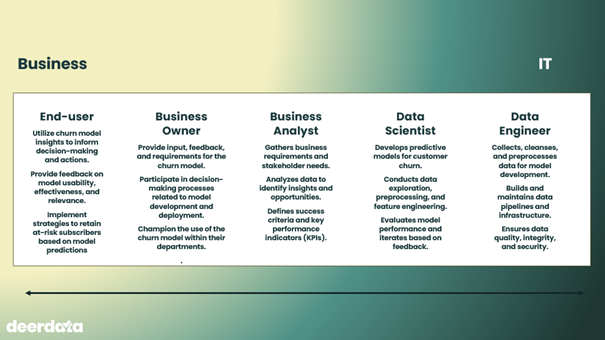
Picture 5: Your dream team to make it happen.
4. Data: The Heartbeat of Your Churn Prediction Model
So you have a great plan, now you just have to get started with the data… great. Easier said than done it turns out. If you have not already worked with your data, this is where you are likely to get stuck. For now, let’s assume you planned ahead, and we have a look at some interesting data points to add to our Deerdata TV Churn model. These are just examples, and some advice is to start with the data you have and not be overly ambitious to start with. Then you will end up just in the waiting line for the right data with nothing delivered in terms of value.
- Subscriber Interactions – how frequently are customers watching Deerdata.se
- Subscription Details – how long have they been customers
- Customer Demographics – how old are customers and where do they live
- Service Interactions – customers complaints, searches on web and app
- External factors – what is our competitors are doing, seasonal factors or economic indicators
By collecting and analyzing these types of data, Deerdata TV can gain valuable insights into subscriber behavior and usage patterns, enabling the development of a robust churn prediction model that can identify at-risk subscribers and inform targeted retention strategies. It’s essential to ensure the quality, accuracy, and completeness of the data to maximize the effectiveness of the customer churn prediction model.
5. The Technical Ecosystem: A Symphony of Systems
I had a colleague a few years ago that complained about the number of systems, requesting IT to slim it down to one system, suggesting we could call it “the System”. Unfortunately, the world is more complex, and a modern IT landscape consists of functions that enable different capabilities that play together. Below is an overview of the capabilities and that are normally involved in the ecosystem of enabling a churn use case and implementing a customer churn prediction model. Here is a short description of the different components. Please note this is a conceptual overview and that there are other ways and alternatives for building this, but this is an example.
- Data source: The starting point for any churn analysis, involving the collection of varied customer data from interactions, transactions, and behaviours.
- Data Foundation: The central home for your data, this involves organizing and structuring the collected data to ensure it’s clean, consistent, and ready for analysis.
- Decisioning: the home to your AI and applying analytical models to the data to identify patterns that indicate a risk of churn.
- Orchestration: time to gather the data and build customer profiles that ensure consistent and personalized experiences across all channels
- Activation: The step where insights are put into action through targeted customer retention strategies and campaigns in different channels.
- Insight: Remember the KPIs and importance of tracking progress? Make sure you have the results of the churn prevention actions to build your future strategies and maybe adjust course.
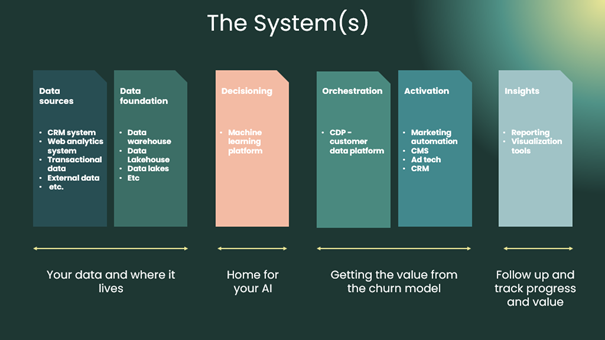
Picture 6: The System(s)
Deer advice: our best wishes on your journey
As we wrap up, we hope you’ve enjoyed this walkthrough and that you can carry something from it into your own journey. Remember, the journey to implementing a churn model is both challenging and rewarding. Our advice is to embrace cross-functional collaboration, prioritize data quality, never lose sight of the value, foster a culture of learning, and most importantly, celebrate every milestone with cake! It’s easy to lose sight of progress when the journey is long. Adding the ’cake KPI’ to your dashboard might just sprinkle some magic into your life, even if AI is fundamentally math! Did you miss the cake KPI check out more here. Now I wish you all the best and lots of cake!
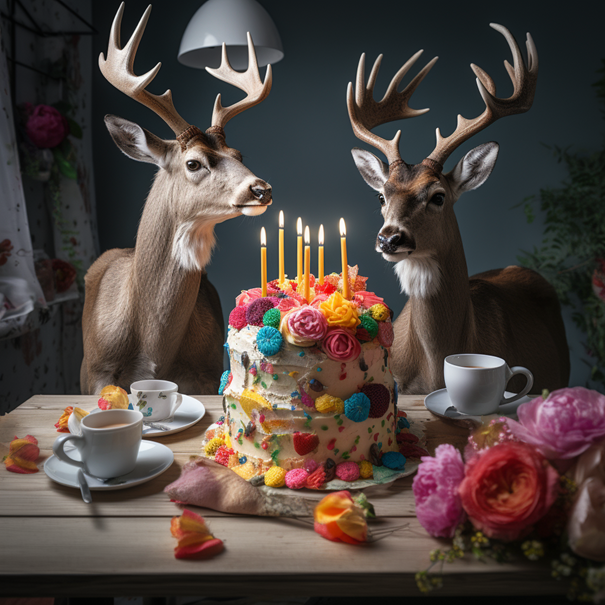
Picture 7: the Cake KPI in action